aisot’s approach to ML for next generation Asset Management
The art of providing insights for next generation Asset Management, from analytics to directly optimized mixed-basket products, is about applying smarter processes to better data. aisot designs and implements AI based portfolio optimization services that allow to extract better results from less but more relevant data sources.
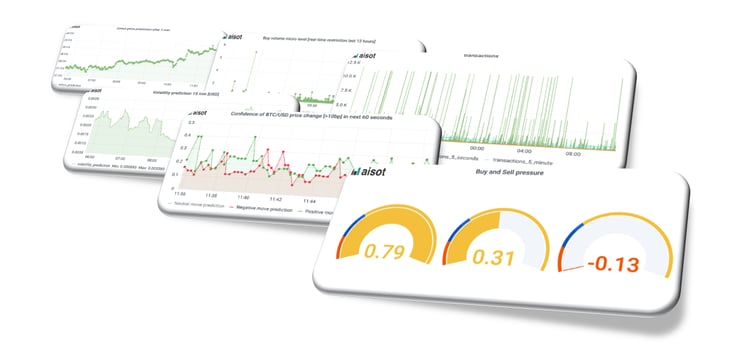
Machine Learning and related buzzwords such as artificial intelligence or quant finance are changing decision making in most areas of financial services. Recent years have brought substantial achievements in the area of machine learning. Considering the high volume, accurate historical records, and quantitative nature of financial services, few industries are better suited for machine learning. There are more use cases of machine learning in finance than ever before, a trend perpetuated by more accessible computing power and more accessible machine learning tools. Machine learning is used to identify anomalies in execution logs, for risk management and fraudulent transaction detection. Elsewhere in financial services, machine learning is leveraged for customer segmentation and support and pricing the derivatives. While those applications are adding real value to the industry, machine learning techniques are the most impactful on the buy-side of things when used for searching signals allowing to now- and forecast a set of patterns. Those include but are not limited to order book pressure, volatility, volumes and price information and trends. Applications range from trading, market making and risk management to direct portfolio optimization.
Impact of information on markets
It is no secret that positive news motivate investors to buy financial products such as stocks or digital assets. Solid earnings reports, announcements of a new product, corporate acquisitions, positive economic indicators and many other events can translate into buying pressure and an increase in prices. The fact that publicly available news have an impact in financial markets is subject of research and studies long before social media and Reddit fueled the hype around trading and crypto currencies. Louis Bachelier researched the subject at the turn of the century from the 19th century into the 20th century. Based on the realization that the information flow available to traders governs dynamics of price returns, new models were introduced such as the sequential information arrival model, news jump dynamics or truncated Levy processes to name a few.
With the rise of the World Wide Web and social media came an ever-increasing abundance of available data, allowing for more detailed studies of the impact of news on financial markets at different time-scales. Big data, coupled together with advancements in machine learning and complex systems research, enabled more efficient analysis of financial data, such as web behaviour data, social media, web search queries, online blogs and other alternative data sources.
Asset allocation optimization
Portfolio management is the art and science of selecting and overseeing a group of investments that meet the long-term financial objectives and risk tolerance of a client, a company, or an institution (Investopedia). In financial economics the efficient-market hypothesis (EMH) states that asset prices reflect all available information. However, market participants are not always following rational patterns but often tend to speculative behavior and irrationality. Even more so in recent months and years, with high levels of volatility due to global events (pandemic), social events (see wallstreetbets, tweets and bitcoin) and developments specific to certain asset classes (digital assets in general). That’s where machine learning techniques come into play. It allows condense large amounts of information into manageable and meaningful insights, or signals, that in return help to minimize risks while maximizing returns.
Machine learning frameworks can be used in several ways for portfolio optimization, such as supervised, unsupervised and reinforcement learning and its sub-frameworks. While most predictions for financial services rely on supervised learning and particularly the framework of regression, the combination and integration of several machine learning frameworks including reinforcement learning and supervised learning enables to decipher and predict a number of variables that are related to volatility and other factors. In fact, asiot integrates all areas of machine learning and combines those with a complex systems approach in order to understand and quantify non-linear interaction patterns of a large number of individual players.
Image: Source
aisot’s approach
Leveraging temporal, rolling and incremental learning and evaluation processes, aisot builds on temporal mixture models in machine learning. Temporal mixture models allow deciphering time-varying effects of order book features on volatility while keeping interpretability. Overall, this method leads to significantly better predictions and a reduction of forecasting errors by up to 50%.
-
Better predictions
aisot dynamically combines different data sources with mixed characteristics in real-time building on temporal mixture models in machine learning. Aisot’s signals outperform a variety of statistical and machine learning baselines as well as industry standards.
-
Reduction of forecasting errors
Numerous research papers by aisot’s co-founders as well as aisot’s work with its clients demonstrate that temporal mixture models reduce forecasting errors by up to 50%. This allows our clients to lower their risks & costs while increasing their revenues.
The results from this approach and the aisot Machine Learning pipeline more in general can either be used to pre-inform certain portfolio optimization steps with services like volatility forecasting for a broad range of asset classes and time frames as well as covariance metrics. And then state-of-the-art Machine Learning can also be used for the actual optimization step itself, e.g. when using a Black Litterman approach.
In summary, the art of providing insights for next generation Asset Management, from analytics to directly optimized mixed-basket products, is always about applying smarter processes to better data. aisot designs and implements AI based portfolio optimization services that allow to extract better results from less but relevant data sources and by combining real-time data features from both market and alternative datasets in a so-called collapsed process.
Informed investment decisions thanks to machine intelligence
aisot signals cover multiple assets classes such as digital assets, forex, commodities or equities and are ideally positioned to optimize and manage multi-asset baskets. aisot’s approach to portfolio optimization is not only 4-5 times more resource efficient than inhouse development but also 3-4 times faster. aisot’s signals allow optimizing portfolios in real-time and can be easily accessed via API and Grafana dashboard. aisot signals are not limited to sentiment analysis but integrate relevant information across the entire news and information spectrum including behaviour data, social media, web search queries, online blogs and other alternative data sources. By providing directly actionable signals for clearly defined time horizons and use cases, aisot goes beyond existing solutions and bridges the last mile from data to trade.